Those clamoring over Greenland’s mineral riches may want to consult Lauren Yeung. A master’s dissertation by the data scientist at London startup Rebel Energy (and former intern at our Institute) shows how a data-driven approach can help in the hunt for Greenland’s untapped copper resources.
Map highlighting the potential presence and distribution of copper in Inglefield Land, Greenland. Source: Lauren Yeung.
The research demonstrates the use of probability-based AI to pinpoint prospecting for copper in Greenland’s Inglefield region and arrives amid growing global attention to the ice-bound island, which harbors an array of minerals that are essential for the energy transition.
Though miners have prospected for copper in Greenland since the 19th century, the pace has accelerated considerably in recent years, as technologies like electric cars fuel demand for copper, which is an essential component in batteries. It may speed up more: The anticipated supply of copper from announced mining projects is expected to meet only 70% of demand for the metal between now and 2035.
Yeung chose to explore the Inglefield region in Greenland’s northwest because of its location away from the cluster of mining underway in the island’s southwest. “I wanted to look somewhere where an AI model may be more useful for mineral prospecting,” she explains.
The research, which she conducted at Durham University, addresses a key challenge in prospecting for minerals: How to separate uncertainty inherent in the use of data-driven mapping from information that can help miners target their exploration.
The challenge has intensified as machine learning techniques become increasingly valuable for exploring locations that have yet to be mapped or for which miners have limited data. The more precise the mapmaking, the more uncertainty that can arise from statistical noise.
To sharpen the prediction, Yeung overlaid remote sensing data on a map representing the copper-rich region in Greenland’s northwest, training the model on images that teach it to infer the location of copper by inferring (and omitting) areas that present high statistical uncertainty. In short, Yeung shows how to make the maps smarter.
The assessments create opportunity for companies that are scouring Greenland and other regions for deposits of copper and other minerals that are important for the transition to a clean-energy economy. They also may help Greenland’s government, which finds itself pursued by suitors that include both the U.S. and China, unlock the island’s mineral resources for the benefit of its own national interest.
Though Yeung has yet to visit Greenland, her research promises to illuminate the way for fellow explorers. “The generated map has the potential to aid targeted exploration and inform investment decisions,” Yeung observes. The research, she notes, “suggests that the majority of uncertainty in the model comes from noise in input data.”
Advancing Mineral Prospectivity Mapping
The study uses a Bayesian convolutional neural network to predict where copper might be found in Inglefield Land, Greenland. It combines geochemical and satellite data to improve mineral exploration. Results show that most uncertainty comes from errors in the data itself, not the model. The generated map could help guide mining exploration and investment, but since data is limited, understanding and managing uncertainty is key to making reliable predictions.
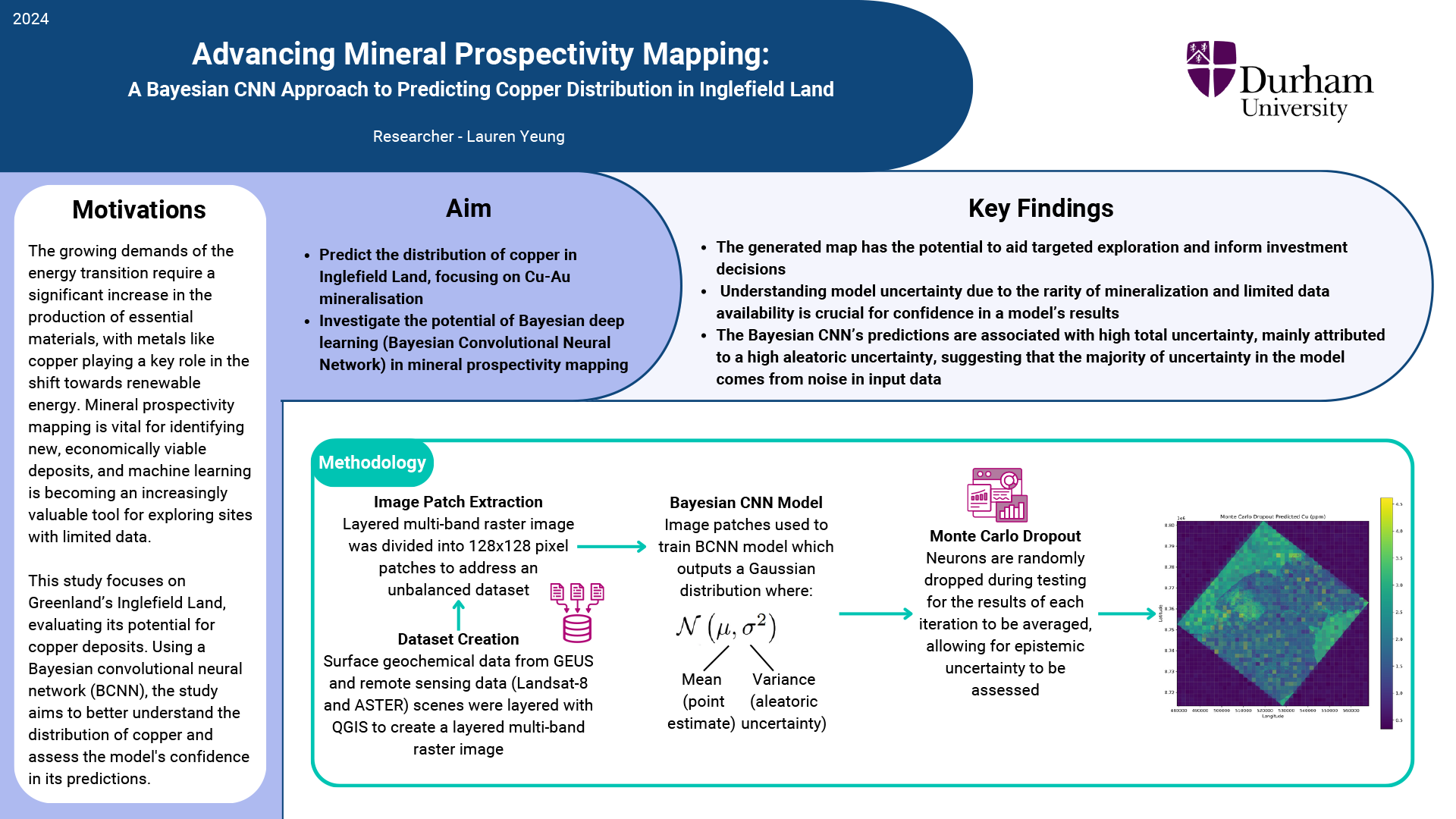